Scientists Build Mathematical Toolkit to Help Battle Cancer
Dino Lirios | | Nov 04, 2014 06:57 AM EST |
(Photo : Vergililus Eremit/Wikipedia) Mathematics formulae
A team has put together a mathematical toolkit combining artificial intelligence and physics that will help in beating cancer.
Man has amassed unimaginable quantities of data. All that data is beginning to become a problem in the fight against cancer. Better and faster gene sequencing techniques mean mountains of new genomic information.
Like Us on Facebook
Within all the noise from the data, there's bound to be some useful information that will assist in their hunt for new and better approaches to beating cancer.
The data problem is not inherent to the fight against cancer alone. It's also evident in astrophysics and climate science as much as it is in medical research.
A group of researchers led by Harvard Medical School's Peter Sorger has found a solution for this conundrum. And it lies outside of medicine.
Sorger and his team have formulated a mathematical toolkit that draws on the techniques of statistical physics and artificial intelligence. This toolkit is capable of identifying the "social networks" of cell proteins.
"[The Cancer Genome Atlas] and similar projects have generated extensive data on the mutational landscape of tumors," Sorger and his team wrote.
"To understand the functional consequences of these mutations, it is necessary to ascertain how they alter the protein-protein interaction networks involved in regulating cellular phenotypes."
He goes on to say the problem with interpreting the data lay in an absence of a unified framework allowing for diverse measurements and the creation of solid models of cancer mutations and their effects.
Soger's work focuses on protein interaction domains (PID), a network that acts as a venue for cell signaling.
The model is based on "statistical mechanics," a way of predicting the likelihood that some system will wind up in a particular state using the thermodynamic energy of a current state.
The new model applies machine learning techniques to the usual principles of statistical machines. Thus, it becomes possible to predict how an individual mutation might propagate through or influence a network.
©2015 Chinatopix All rights reserved. Do not reproduce without permission
EDITOR'S PICKS
-
Did the Trump administration just announce plans for a trade war with ‘hostile’ China and Russia?
-
US Senate passes Taiwan travel bill slammed by China
-
As Yan Sihong’s family grieves, here are other Chinese students who went missing abroad. Some have never been found
-
Beijing blasts Western critics who ‘smear China’ with the term sharp power
-
China Envoy Seeks to Defuse Tensions With U.S. as a Trade War Brews
-
Singapore's Deputy PM Provides Bitcoin Vote of Confidence Amid China's Blanket Bans
-
China warns investors over risks in overseas virtual currency trading
-
Chinese government most trustworthy: survey
-
Kashima Antlers On Course For Back-To-Back Titles
MOST POPULAR
LATEST NEWS
Zhou Yongkang: China's Former Security Chief Sentenced to Life in Prison
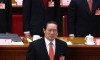
China's former Chief of the Ministry of Public Security, Zhou Yongkang, has been given a life sentence after he was found guilty of abusing his office, bribery and deliberately ... Full Article
TRENDING STORY
China Pork Prices Expected to Stabilize As The Supplies Recover
Elephone P9000 Smartphone is now on Sale on Amazon India
There's a Big Chance Cliffhangers Won't Still Be Resolved When Grey's Anatomy Season 13 Returns
Supreme Court Ruled on Samsung vs Apple Dispute for Patent Infringement
Microsoft Surface Pro 5 Rumors and Release Date: What is the Latest?